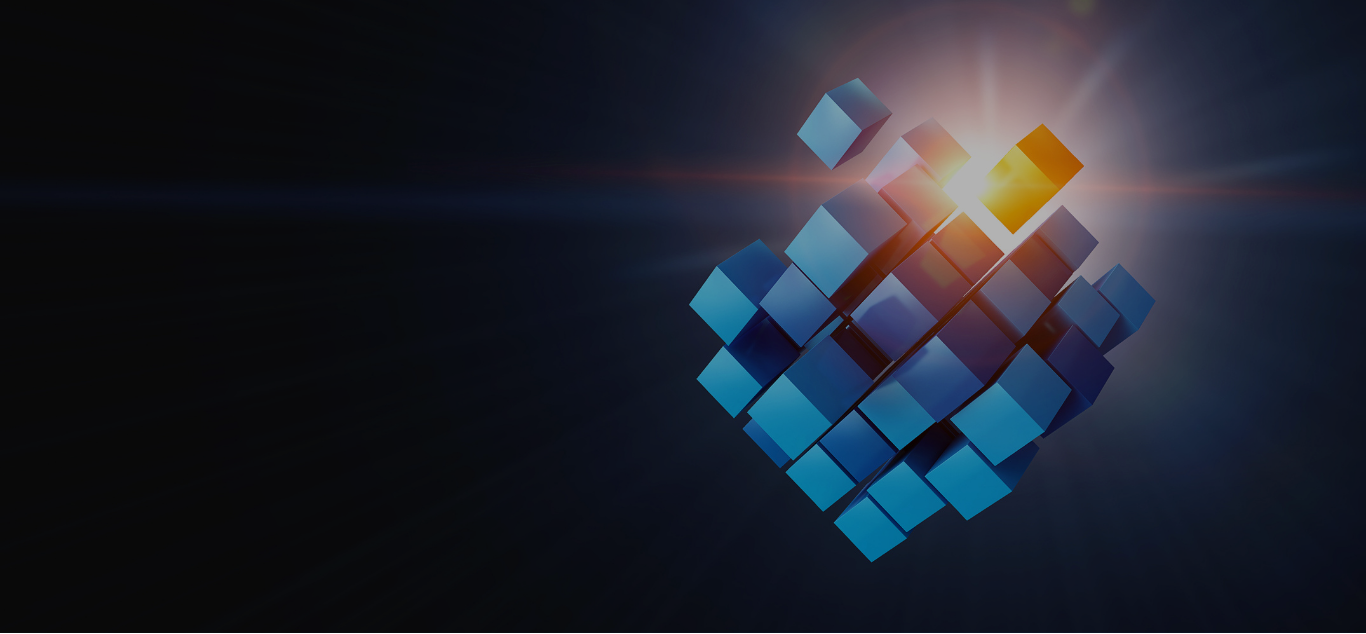
The Cosmo Tech AI-Simulation platform provides a range of tools and methods for simulating complex systems, addressing business needs. Part of the Cosmo Tech CoMETS library, it includes experiments like uncertainty and sensitivity analysis, parameter sweep, and reinforcement learning. These tools enable precise modeling of real scenarios, revealing insights, optimizing results, and improving decision-making.
Uncertainty analysis evaluates the impact of input uncertainties on model outputs, offering:
In demand forecasting, uncertainty analysis helps improve prediction accuracy by examining variables like market trends, seasonal patterns, and economic fluctuations. It enables creating probability distributions for various demand scenarios, optimizing inventory, refining production schedules, and better resource allocation, thus reducing risks related to stockouts and surplus inventory.
This experiment helps understand how input changes affect model outcomes, pinpointing key influential variables. It simplifies understanding their impact:
Sensitivity analysis is key for data modelers and risk managers in evaluating how risk scenarios like delivery delays and supplier issues affect supply chain performance. It allows them to measure the sensitivity of performance indicators, identify critical vulnerabilities, prioritize risk mitigation, and allocate resources to improve supply chain resilience.
The parameter sweep offers a powerful approach to thoroughly explore a model’s entire parameter spectrum.
Asset Performance Analysis: The Parameter Sweep experiment helps analyze asset performance across various conditions in asset-intensive sectors. By adjusting maintenance, equipment, and strategy parameters, data modelers and asset managers can evaluate their impact on performance metrics such as costs, downtime, and capacity. This identifies strategies to enhance performance and reduce downtime.
The CoMETS library enables reinforcement learning environments for Cosmo Tech simulators with:
Sustainability and Energy Integration: Reinforcement learning and AI Simulation optimize energy source selection to reduce costs and CO2 emissions. Agents explore energy combinations, adjusting strategies based on production, emissions, and market prices. This creates an autonomous system that adapts to changes, optimizing energy mixes for sustainable decision-making and energy transition.